Artificial Intelligence (AI) applications are at our fingertips every day – think Siri, Alexa, Netflix recommendations, and spam filters. Most AI algorithms use large quantities of data to learn about human behavior (called machine learning). Machine learning is increasingly used in decision making – examples include criminal justice, credit/loans, recruitment, education, and clinical diagnosis. These automated systems raise questions about bias, fairness, privacy, and transparency.
To understand these complex questions, we need to look under the hood of the underlying data used by machine learning algorithms.
Data-driven systems still rely on human judgment to:
1. Sort and categorize data
2. Define the characteristics of the data, and
3. Qualify attributes related to the data
This can lead to decision-making processes that are themselves objective; however, those decisions are based on data prone to very human subjectivity and errors. In fact, it is theorized that there are around 188 cognitive biases that impact how we receive and categorize information. (“Bias” refers to any decision outcome that can lead to unintended discrimination and inequality.)
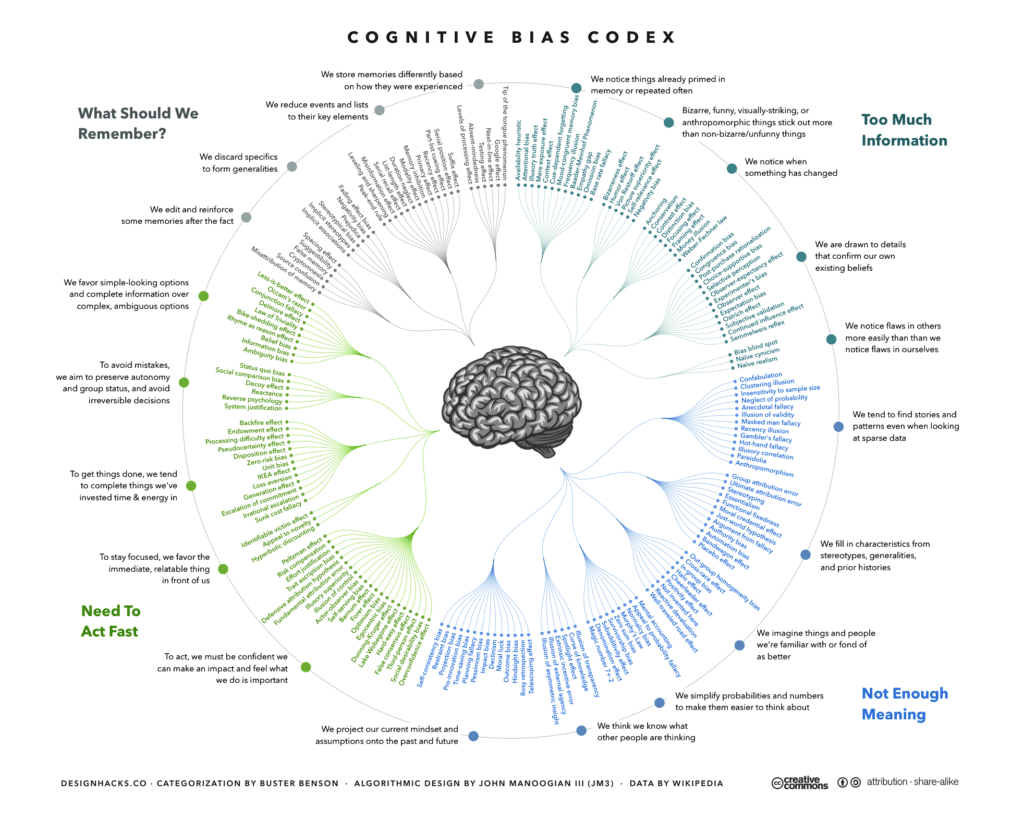
This can be overwhelming to look at when we are building AI systems. However, we can start by analyzing these systems over five key areas to help us with implementing human-centric AI. Let us focus on five critical areas of bias and how we can measure our AI systems against these:
1 ) Fairness: Fairness is an essential component of an equitable society. We have heard of unfair algorithms where facial recognition software suggests dark-skinned individuals are prone to crime or Southeast Asians blink more. There are many ways to create safeguards against this to create fair and just AI systems:
- Obtaining feedback/information from a diverse team of individuals
- Analyzing the data for inclusive representation (e.g., training data from all ages or all geographies depending on the problem you are solving)
- Exploring data limitations (for example, ensuring that you have obtained images of a disease from a diverse geographic region and not just one area.)
2 ) Transparency: AI is often viewed as a “black box.” We wonder about “how and why the algorithm” made a particular recommendation. Auditability of the system is crucial in industries like healthcare and finance, and is achieved through:
- Incorporating explainability of the models by revealing the factors/attributes of the decision-making algorithm. The granularity of the explanation will depend on the use case, but regardless, the system should withstand scrutiny if the need arises
- Announcing chatbots or voice-operated bots like Google’s Duplex upfront, which increases the transparency of automated systems and ensures that people trust and adopt them
3 ) Responsibility: Responsible AI is ethically designed for the well-being of people and society at large. Building responsible AI systems requires questioning the intent every step of the way through:
Using human-centric design techniques like IDEO
- Building AI systems to adhere to internationally recognized human rights
- Questioning and cataloguing the impact of the system on external entities (e.g., passing data to apps like Facebook)
- Tweaking algorithms where necessary to ensure that all demographics are represented fairly in outcomes
- Being prepared for secondary effects (e.g. who is liable in a car crash caused by a self-driving car?)
4 ) Privacy: In today’s digital world, our data is knowingly or unknowingly used by various AI applications. With this in mind, safeguarding privacy in AI means:
- Designing software that provides options for everyone to control their data (e.g. settings or preferences)
- Creating terms and conditions that include a list of who has the right to access, share, and benefit from their data and the insights it provides
- Informing stakeholders about the consequences of a data breach or the resale of data to third parties
5 ) Accountability: As legislation catches up to the effects of AI,we need systems that have accountability built-in. Compliance with legalities is not sufficient to track the use of AI systems, data, or any personal information on the internet. We must broaden standards to involve the following:
- Using aggregated data instead of individual data (e.g. deleting personal data and using grouped data – such as people within a specific location or demographic)
- Designing for various levels of autonomy (depending on the use case, the AI system can give a recommendation, but the ultimate decision lies with a human)
- Analyzing how existing regulations apply to AI systems and identify gaps, if any
- Including a committee to discuss AI governance issues
When used effectively, AI can boost productivity, economy, and social benefits. However, we need to build intelligent systems that address the current challenges of bias through human-centric design techniques. This can be achieved by evaluating whether algorithms are fair, offering explainability and transparency, making data secure and safe, and lastly, showing accountability. The ability to confront the risks associated with AI misuse and to take steps to minimize bias is within our hands.
Integrity Management Services, Inc. (IntegrityM) is a certified
women-owned small business,ISO 9001:2015 certified, and FISMA compliant
organization. IntegrityM was created to support the program integrity efforts
of federal and state government programs, as well as private sector
organizations. IntegrityM provides experience and expertise to government
programs and private businesses supporting government programs. Results are
achieved through analysis and support services such as statistical and data
analysis, software solutions, compliance, audit, investigation, and
medical review.